KITE improves the efficiency of electric motors in vehicles by automating the design process, thereby reducing costs and emissions.

The customer
The challenge

Project duration: 01.09.2021 - 31.08.2024
Electric motors have become an indispensable part of the transportation revolution. They have established themselves in both the private and commercial sectors. The design of these motors is increasingly focusing on the consumption of raw materials. The creation of engine topologies is a highly complex process that depends on many factors, such as the desired power class of the engine. Currently, the technology is based on physical simulations that must be manually configured and repeated for changing requirements.
In KITE, methods are being jointly developed to modernize these processes with the help of AI and make them more flexible. The focus is on the interpretability and physical plausibility of the generated results in order to be able to optimally tie in with existing expert knowledge. Finally, the developed AI methods are applied to data generated specifically in the project in order to find a solution topology that is as optimal as possible. In the final step, this is manufactured and tested by means of a physical prototype.
Solution

Results & effects
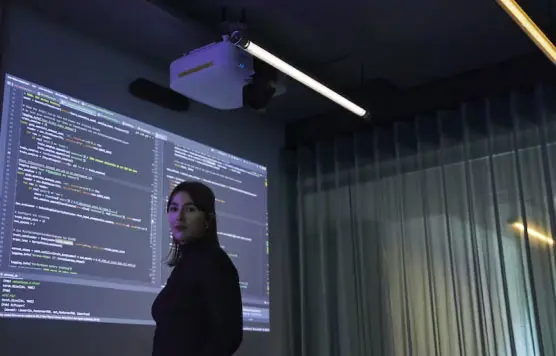
Merantix Momentum is conducting research in the KITE project on novel AI methods to speed up the laborious simulation process for creating engine topologies and make it more flexible. The AI models developed can suggest previously unknown, highly efficient topologies that were not previously covered by simulation. Using suitable optimization algorithms, pre-trained AI models can be used to search their solution space and find an ideal engine topology with respect to various criteria.
In order to explain the AI methods used, complete neural networks are employed in KITE, which provide information about learned correlations and serve as a basis for discussions with domain experts. For the most data-efficient approach possible, Merantix Momentum develops methods for data augmentation here and uses them in a targeted manner in combination with model architectures adapted to the domain, such as Graph Neural Networks (GNNs) .