AI research
● for the solutions of the future
We apply transformative AI research to real-world challenges, collaborating closely with partners from academia, business, and industry.
Our research partners:
Our mission
What drives our research?
01
AI for the future
We research for the future viability of our partners
- We conduct cutting-edge research to develop new solutions to unsolved problems with our partners and customers
- Horizontal application of machine learning in real-world challenges from all sectors of business and society.
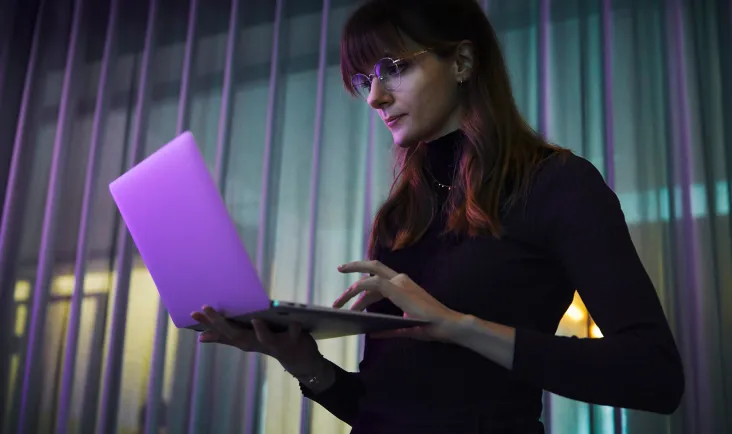
02
The potential of data
We open up unlabeled data with methodology
- Impactful research outcomes through a combination of data-centric approaches and methodological excellence
- Integrate results into established and emerging systems through experience in scaling and operationalizing AI

Our approach
First-class research is created in partnership.
In all our research projects, we deal with state-of-the-art machine learning methods and apply them agnostically in all relevant application areas. During the operational project phase, we staff the projects with a diverse team of experts in research, machine learning engineering, and project management.
As industry-independent developers, we strive to develop generalizable methods that we can easily apply in other domains. We leverage our broad background in methodologies and industries to quickly adapt and deliver meaningful results for new challenges.
As industry-independent developers, we strive to develop generalizable methods that we can easily apply in other domains. We leverage our broad background in methodologies and industries to quickly adapt and deliver meaningful results for new challenges.
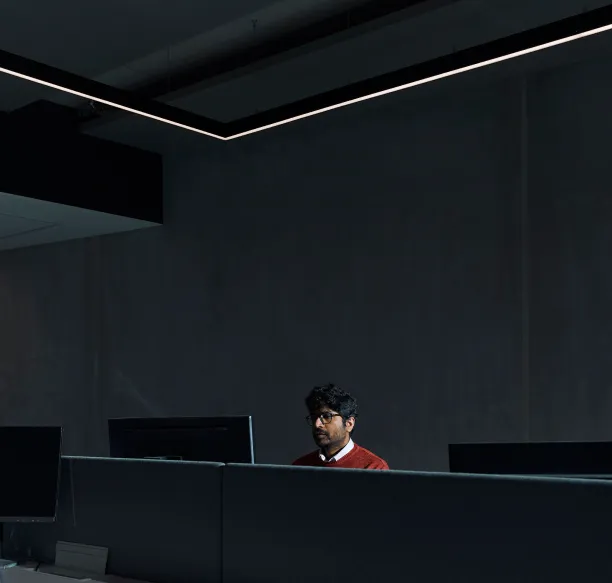
At Merantix Momentum, we initiate and participate in a variety of collaborative partnerships across different sectors and funding programs. Our dedicated research management team has more than 30 years of experience in designing and managing research projects, with a particular focus on German and EU-funded collaborative research projects.
We carefully tailor our engagement with a new research project by considering its unique challenge and all partners involved. Our team is able to establish effective consortia and create compelling proposal documents that ensure successful project funding.
We carefully tailor our engagement with a new research project by considering its unique challenge and all partners involved. Our team is able to establish effective consortia and create compelling proposal documents that ensure successful project funding.
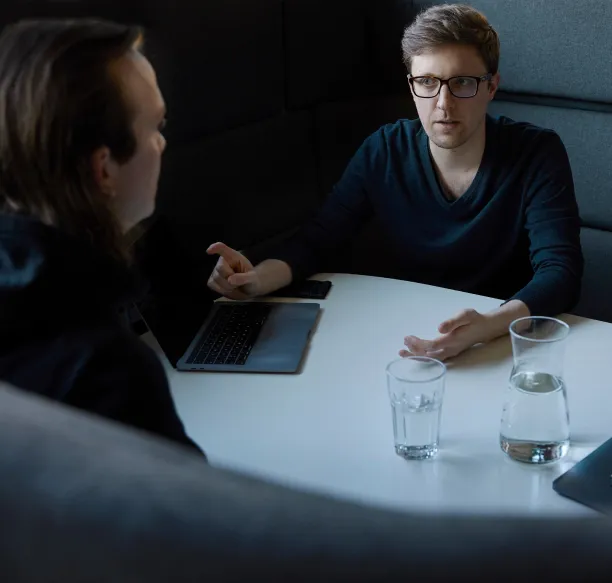
We see collaboration as the foundation for our success. As part of the AI Campus ecosystem in Berlin, connecting bright minds and new ideas is essential to our identity. This self-image as an innovative and forward-looking team player is also reflected in the campus, which is an ideal place for stimulating project meetings and inspiring events.
Our goal is to contribute to the scientific community by publishing our results in research papers and presenting them at conferences. The driving motivation here is always to generate added value for the entire research community and thus advance research in AI and machine learning as a whole.
Our goal is to contribute to the scientific community by publishing our results in research papers and presenting them at conferences. The driving motivation here is always to generate added value for the entire research community and thus advance research in AI and machine learning as a whole.


Our research projects
What we are currently working on
Our research pushes boundaries. We bring our excellence in machine learning to pioneering projects that span all industrial domains to solve real-world challenges.
In our partnerships, we explore breakthrough solutions, discover new approaches, and apply them to known and emerging domains. We create the conditions for transformative research.
In our partnerships, we explore breakthrough solutions, discover new approaches, and apply them to known and emerging domains. We create the conditions for transformative research.
Publications
SECREDAS: Safe and (Cyber-)Secure Cooperative and Automated Mobility (2023)
This is some text inside of a div block.
This is some text inside of a div block.Sebastian Gerres
IFAC 2023 - Full PDF at arxiv.org
Uncovering the Inner Workings of STEGO for Safe Unsupervised Semantic Segmentation (2023).
This is some text inside of a div block.
This is some text inside of a div block.Alexander Koenig, Maximilian Schambach, Johannes S. Otterbach
CVPR 2023 - Full PDF at arxiv.org
Interpretable Reinforcement Learning via Neural Additive Models for Inventory Management (2023).
This is some text inside of a div block.
This is some text inside of a div block.Julien Siems, Maximilian Schambach, Sebastian Schulze, Johannes S. Otterbach
ICLR 2023 - Full PDF at arxiv.org
Learning the Solution Operator of Boundary Value Problems using Graph Neural Networks (2022).
This is some text inside of a div block.
This is some text inside of a div block.Winfried Lötzsch, Simon Ohler, Johannes S. Otterbach
ICML 2022 - Full PDF at arxiv.org
Scalable Flow Optimization for Small Satellite Networks using Benders Decomposition (2022).
This is some text inside of a div block.
This is some text inside of a div block.Olga Kondrateva, Stefan Dietzel, Björn Scheuermann
IEEE WoWMoM 2022 - Full PDF at ieee.org
Towards Learning Self-Organized Criticality of Rydberg Atoms using Graph Neural Networks (2022).
This is some text inside of a div block.
This is some text inside of a div block.Simon Ohler, Daniel Steven Brady, Winfried Lötzsch, Michael Fleischhauer, Johannes Otterbach
AI4Science Workshop at ICML 2022 - Full PDF at arxiv.org
Auto-Compressing Subset Pruning for Semantic Image Segmentation (2022)
This is some text inside of a div block.
This is some text inside of a div block.Konstantin Ditschuneit, Johannes S. Otterbach
GCPR 2022 - Full PDF at arxiv.org
MEAL: Manifold Embedding-based Active Learning (2021).
This is some text inside of a div block.
This is some text inside of a div block.Deepthi Sreenivasaiah, Johannes Otterbach, Thomas Wollmann
CPVR 2021 - Full PDF at arxiv.org
DAAIN: Detection of Anomalous and Adversarial Input using Normalizing Flows (2021).
This is some text inside of a div block.
This is some text inside of a div block.Samuel von Baußnern, Johannes Otterbach, Adrian Loy, Mathieu Salzmann, Thomas Wollmann
Full PDF at arxiv.org
Chameleon: A Semi-AutoML framework targeting quick and scalable development and deployment of production-ready ML systems for SMEs (2021)
This is some text inside of a div block.
This is some text inside of a div block.Johannes Otterbach, Thomas Wollmann
Full PDF at arxiv.org
Contact us
- Development of AI solutions in research projects in all industries
- Integration of machine learning in established and emerging systems
- Many years of experience in public and private consortium research
Thank you! Your submission has been received!
Oops! Something went wrong while submitting the form.
We would like to get to know you!
Start your AI journey with us now
Subscribe now to the Merantix Momentum Newsletter.
Thank you! Your submission has been received!
Oops! Something went wrong while submitting the form.